Difference between revisions of "Stone doubling algorithm for the LU decomposition of a tridiagonal matrix"
[unchecked revision] | [unchecked revision] |
Line 82: | Line 82: | ||
<math>p^{new}_i = p_i p_{i-1}, r^{new}_i = p_i r_{i-1} + r_i</math>; at the other steps, they are updated using the formulas <math>p^{new}_i = p_i p_{j}+r_i p_{j-1}, r^{new}_i = p_i r_{j} + r_i r_{j-1}</math>. | <math>p^{new}_i = p_i p_{i-1}, r^{new}_i = p_i r_{i-1} + r_i</math>; at the other steps, they are updated using the formulas <math>p^{new}_i = p_i p_{j}+r_i p_{j-1}, r^{new}_i = p_i r_{j} + r_i r_{j-1}</math>. | ||
− | + | Then, again, <math>2^{k-1}</math> numbers are skipped, and, for the next <math>2^{k-1}</math> numbers, calculations similar to those above are performed, and so on until the range of <math>i</math> from 2 to <math>n</math> is run out. | |
− | + | The above steps are repeated until it occurs that all the values of <math>i</math> from 2 to <math>n</math> should be skipped. After that, one calculates | |
− | + | <math>q_i = p_i a_{11} + r_i</math> for all <math>i</math> from 1 to <math>n</math>, then <math>u_{ii} = q_i/q_{i-1}</math> and <math>l_{ii-1} = a_{ii-1}/u_{i-1i-1}</math> for all <math>i</math> from 2 to <math>n</math>. | |
− | <math>q_i = p_i a_{11} + r_i</math> | ||
=== Computational kernel of the algorithm === | === Computational kernel of the algorithm === |
Revision as of 12:50, 9 March 2018
Stone doubling algorithm for the LU decomposition of tridiagonal matrices | |
Sequential algorithm | |
Serial complexity | [math]3(n-1)\lceil \log_2 (n-1) \rceil + o((n-1)\lceil \log_2 (n-1) \rceil)[/math] |
Input data | [math]3n-2[/math] |
Output data | [math]2n-1[/math] |
Parallel algorithm | |
Parallel form height | [math]2 \lceil \log_2 (n-1) \rceil + 3[/math] |
Parallel form width | [math]n[/math] |
Main author: Alexey Frolov
Contents
- 1 Properties and structure of the algorithm
- 1.1 General description of the algorithm
- 1.2 Mathematical description of the algorithm
- 1.3 Computational kernel of the algorithm
- 1.4 Macro structure of the algorithm
- 1.5 Implementation scheme of the serial algorithm
- 1.6 Serial complexity of the algorithm
- 1.7 Information graph
- 1.8 Parallelization resource of the algorithm
- 1.9 Input and output data of the algorithm
- 1.10 Properties of the algorithm
- 2 Software implementation of the algorithm
- 2.1 Implementation peculiarities of the serial algorithm
- 2.2 Locality of data and computations
- 2.3 Possible methods and considerations for parallel implementation of the algorithm
- 2.4 Scalability of the algorithm and its implementations
- 2.5 Dynamic characteristics and efficiency of the algorithm implementation
- 2.6 Conclusions for different classes of computer architecture
- 2.7 Existing implementations of the algorithm
- 3 References
1 Properties and structure of the algorithm
1.1 General description of the algorithm
The Stone doubling algorithm for the LU decomposition of tri-diagonal matrices is a part of the Stone doubling algorithm for solving SLAEs [1][2] of the form [math]Ax = b[/math], where
- [math] A = \begin{bmatrix} a_{11} & a_{12} & 0 & \cdots & \cdots & 0 \\ a_{21} & a_{22} & a_{23}& \cdots & \cdots & 0 \\ 0 & a_{32} & a_{33} & \cdots & \cdots & 0 \\ \vdots & \vdots & \ddots & \ddots & \ddots & 0 \\ 0 & \cdots & \cdots & a_{n-1 n-2} & a_{n-1 n-1} & a_{n-1 n} \\ 0 & \cdots & \cdots & 0 & a_{n n-1} & a_{n n} \\ \end{bmatrix}, x = \begin{bmatrix} x_{1} \\ x_{2} \\ \vdots \\ x_{n} \\ \end{bmatrix}, b = \begin{bmatrix} b_{1} \\ b_{2} \\ \vdots \\ b_{n} \\ \end{bmatrix} [/math]
The Stone doubling algorithm was first proposed in early 70's of 20-th century [3] as an alternative to other parallel algorithms for solving tri-diagonal SLAEs such as, for instance, the cyclic reduction method.
Here, we consider the first part of the algorithm that concerns the [math]LU[/math] decomposition. The aim is to represent the matrix
- [math] A = \begin{bmatrix} a_{11} & a_{12} & 0 & \cdots & \cdots & 0 \\ a_{21} & a_{22} & a_{23}& \cdots & \cdots & 0 \\ 0 & a_{32} & a_{33} & \cdots & \cdots & 0 \\ \vdots & \vdots & \ddots & \ddots & \ddots & 0 \\ 0 & \cdots & \cdots & a_{n-1 n-2} & a_{n-1 n-1} & a_{n-1 n} \\ 0 & \cdots & \cdots & 0 & a_{n n-1} & a_{n n} \\ \end{bmatrix} [/math]
as the product of the matrices
- [math] L = \begin{bmatrix} 1 & 0 & 0 & \cdots & \cdots & 0 \\ l_{21} & 1 & 0 & \cdots & \cdots & 0 \\ 0 & l_{32} & 1 & \cdots & \cdots & 0 \\ \vdots & \vdots & \ddots & \ddots & \ddots & 0 \\ 0 & \cdots & \cdots & l_{n-1 n-2} & 1 & 0 \\ 0 & \cdots & \cdots & 0 & l_{n n-1} & 1 \\ \end{bmatrix} [/math]
and
- [math] U = \begin{bmatrix} u_{11} & u_{12} & 0 & \cdots & \cdots & 0 \\ 0 & u_{22} & u_{23}& \cdots & \cdots & 0 \\ 0 & 0 & u_{33} & \cdots & \cdots & 0 \\ \vdots & \vdots & \ddots & \ddots & \ddots & 0 \\ 0 & \cdots & \cdots & 0 & u_{n-1 n-1} & u_{n-1 n} \\ 0 & \cdots & \cdots & 0 & 0 & u_{n n} \\ \end{bmatrix} [/math]
An important point is the fact that, in exact arithmetic, the Stone doubling algorithm finds the same decomposition as that in the compact scheme of Gaussian elimination.
Theoretically, the Stone method is based on the following considerations. Define the values [math]q_i[/math] by the formulas [math]q_0 = 1[/math], [math]q_1 = a_{11}[/math], and [math]q_i = a_{ii} q_{i-1} - a_{ii-1}a_{i-1i} q_{i-2}[/math]. As soon as all the [math]q_i[/math] are calculated, one can easily find all the entries [math]u_{ii} = q_i/q_{i-1}[/math] and [math]l_{ii-1} = a_{ii-1}/u_{i-1i-1}[/math]. The entries [math]u_{ii+1} = a_{ii+1}[/math] need not be calculated.
When calculating the values [math]q_i[/math], one uses the associativity of matrix multiplication. The recursion relation [math]q_i = a_{ii} q_{i-1} - a_{ii-1}a_{i-1i} q_{i-2}[/math] implies the matrix equality
- [math] \begin{bmatrix} q_i \\ q_{i-1} \\ \end{bmatrix} = \begin{bmatrix} a_{ii} & -a_{ii-1}a_{i-1i} \\ 1 & 0 \\ \end{bmatrix} \begin{bmatrix} q_{i-1} \\ q_{i-2} \\ \end{bmatrix} = T_i \begin{bmatrix} q_{i-1} \\ q_{i-2} \\ \end{bmatrix} = T_i T_{i-1}...T_2 \begin{bmatrix} q_{1} \\ q_{0} \\ \end{bmatrix} = T_i T_{i-1}...T_2 \begin{bmatrix} a_{11} \\ 1 \\ \end{bmatrix} [/math]
Matrix products [math]T_i T_{i-1}...T_2[/math] are calculated using the parallel doubling scheme. Note that the second row of each matrix [math]T_i[/math] is identical to the first row of the identity matrix. It follows that the second row of the product [math]T_i T_{i-1}...T_k[/math] is identical to the first row of the product [math]T_{i-1}...T_k[/math], which makes it possible to halve the number of arithmetic operations compared to the analogous product of general matrices of the same order .
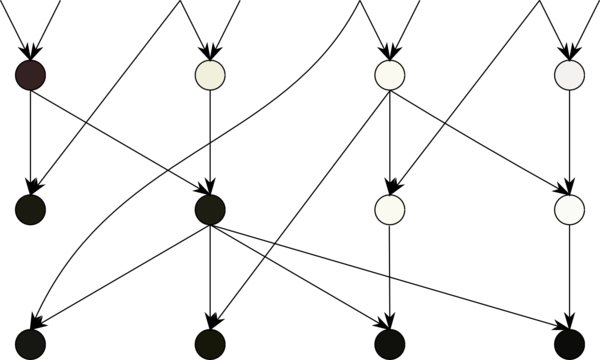
1.2 Mathematical description of the algorithm
First, for all [math]i[/math] from 2 to [math]n[/math], we set
[math]p_i = a_{ii}, r_i = -a_{ii-1}a_{i-1i}[/math],
and
[math]p_1 = 0, r_1 = 1[/math].
At step [math]k[/math], the calculations are conducted as follows:
Beginning from [math]i[/math] = 2, skip [math]2^{k-1}[/math] numbers; for the next [math]2^{k-1}[/math] numbers, set [math]j[/math] equal to the nearest smaller integer of the form [math]j = 1 + 2^{k} m [/math].
At the first step, the values [math]p_i, r_i[/math] are updated by the formulas [math]p^{new}_i = p_i p_{i-1}, r^{new}_i = p_i r_{i-1} + r_i[/math]; at the other steps, they are updated using the formulas [math]p^{new}_i = p_i p_{j}+r_i p_{j-1}, r^{new}_i = p_i r_{j} + r_i r_{j-1}[/math].
Then, again, [math]2^{k-1}[/math] numbers are skipped, and, for the next [math]2^{k-1}[/math] numbers, calculations similar to those above are performed, and so on until the range of [math]i[/math] from 2 to [math]n[/math] is run out.
The above steps are repeated until it occurs that all the values of [math]i[/math] from 2 to [math]n[/math] should be skipped. After that, one calculates [math]q_i = p_i a_{11} + r_i[/math] for all [math]i[/math] from 1 to [math]n[/math], then [math]u_{ii} = q_i/q_{i-1}[/math] and [math]l_{ii-1} = a_{ii-1}/u_{i-1i-1}[/math] for all [math]i[/math] from 2 to [math]n[/math].
1.3 Computational kernel of the algorithm
Вычислительное ядро алгоритма составляют в основной части операции типа [math]ab+cd[/math] и [math]ab+c[/math], с небольшой добавкой отдельных умножений и делений. Изолированные длинные ветви вычислений отсутствуют.
1.4 Macro structure of the algorithm
На макроуровне можно выделить такие 4 макрооперации: вычисление элементов матриц [math]T_i[/math], вычисление сдваиванием произведений матриц [math]T_{i}[/math], вычисление результатов.
1.5 Implementation scheme of the serial algorithm
Метод Стоуна изначально спроектирован для параллельного исполнения, поскольку является по отношению к, например, классической прогонке, алгоритмом с избыточными вычислениями. Смысла в его последовательной реализации нет ещё и из-за того, что он неустойчив.
1.6 Serial complexity of the algorithm
Для полного выполнения алгоритма Стоуна и получения разложения трёхдиагональной матрицы на две двудиагональные нужно выполнить:
- [math]2n-2[/math] делений,
- [math]2(n-1)\lceil \log_2 (n-1) \rceil + o((n-1)\lceil \log_2 (n-1) \rceil)[/math] умножений,
- [math](n-1)\lceil \log_2 (n-1) \rceil +o((n-1)\lceil \log_2 (n-1) \rceil)[/math] сложений.
Поэтому алгоритм должен быть отнесён к алгоритмам линейно-логарифмической сложности по количеству последовательных операций.
1.7 Information graph
Как уже отмечено, макроструктура алгоритма состоит из 4 частей.
В первой части производится вычисление недиагональных элементов матриц [math]T_{i}[/math].
Во второй части - вычисление сдваиванием произведений матриц [math]T_{i}[/math], оно показано на рисунке 1 и использует результаты 1й части.
В третьей, последней, части - вычисление результатов, с использованием результатов 2й части.
Внутренние графы 1й и 3й частей - пусты, их операции в зависимости только со 2й частью.
1.8 Parallelization resource of the algorithm
На критическом пути алгоритма Стоуна для LU-разложения трёхдиагональной матрицы нужно выполнить:
- [math]1[/math] ярус делений,
- [math]\lceil \log_2 (n-1) \rceil+1[/math] ярусов умножений,
- [math]\lceil \log_2 (n-1) \rceil+1[/math] ярусов сложений.
Поэтому алгоритм должен быть отнесён к алгоритмам логарифмической сложности по количеству последовательных операций. Ширина яруса равна [math]n[/math], поэтому алгоритм должен быть отнесён к алгоритмам линейной сложности по ширине ярусов.
1.9 Input and output data of the algorithm
Входные данные: трёхдиагональная матрица [math]A[/math] (элементы [math]a_{ij}[/math]).
Объём входных данных: [math]3n-2[/math]. В разных реализациях размещение для экономии хранения может быть выполнено разным образом. Например, каждая диагональ может быть строкой массива.
Выходные данные: нижняя двухдиагональная матрица [math]L[/math] (элементы [math]l_{ij}[/math], причём [math]l_{ii}=1[/math]) и верхняя двухдиагональная матрица [math]U[/math] (элементы [math]u_{ij}[/math]).
Объём выходных данных: формально [math]3n-2[/math]. Однако благодаря совпадению [math]n[/math] входных и выходных данных реально вычисляется лишь [math]2n-2[/math] элементов.
1.10 Properties of the algorithm
Соотношение последовательной и параллельной сложности, как хорошо видно, равно [math]O(n)[/math].
При этом вычислительная мощность алгоритма как отношение числа операций к суммарному объему входных и выходных данных является логарифмической.
Алгоритм в рамках выбранной версии полностью детерминирован.
Устойчивость алгоритма остаётся только при условии невозрастания ведущих главных миноров матрицы. При росте миноров область устойчивости алгоритма очень мала.
2 Software implementation of the algorithm
2.1 Implementation peculiarities of the serial algorithm
Из-за большой избыточности вычислений алгоритм сдваивания Стоуна никогда не предназначался для последовательной реализации. После обнаружения его неустойчивости стало ясно, что и в будущем он не будет реализован на последовательных архитектурах.
2.2 Locality of data and computations
Хотя исследование локальности и не имеет смысла из-за неустойчивости, можно сказать, что, как видно по графу алгоритма, ряд дуг длинны как по времени (по различию номеров ярусов операций, являющихся началом и концом дуги), так и по пространству (исключением является только размещение в гиперкубе, физически невозможное). Эта неустранимая нелокальность должна тормозить исполнение алгоритма. Реальное же исследование последовательного кода на обращения в память проводить бессмысленно, поскольку последовательный код не применяется и не будет применяться никем.
2.3 Possible methods and considerations for parallel implementation of the algorithm
Из-за неустойчивости алгоритма любые способы реализации теряют смысл.
2.4 Scalability of the algorithm and its implementations
Из-за неустойчивости алгоритма не имеют смысла любые разговоры о масштабируемости любых его реализаций.
2.5 Dynamic characteristics and efficiency of the algorithm implementation
Из-за неустойчивости алгоритма не имеют смысла любые замеры эффективности любых его реализаций и их динамических характеристик. Кроме того, из-за избыточности вычислений ясно, что реальная эффективность будет существенно ограничена даже при малых размерах задач, где неустойчивость ещё не сказывается.
2.6 Conclusions for different classes of computer architecture
На области относительно приемлемых ошибок (малые размеры задач) у метода всё равно нет шансов на нормальную реализацию - быстрее будет СЛАУ прогонкой на обычной персоналке решить.
2.7 Existing implementations of the algorithm
Из-за неустойчивости алгоритма его не используют на практике, поэтому планировавшаяся в исходной публикации[3] замена более популярной циклической редукции не удалась.
3 References
- ↑ Воеводин В.В. Вычислительные основы линейной алгебры. М.: Наука, 1977.
- ↑ Воеводин В.В., Кузнецов Ю.А. Матрицы и вычисления. М.: Наука, 1984.
- ↑ 3.0 3.1 Stone H.S. An Efficient Parallel Algorithm for the Solution of a Tridiagonal Linear System of Equations // J. ACM, Vol. 20, No. 1 (Jan. 1973), P. 27-38.
Категория:Метод сдваивания Категория:Неустойчивые_параллельные_методы Категория:Алгоритмы с избыточными вычислениями Категория:Разложения матриц