Difference between revisions of "Poisson equation, solving with DFT"
[unchecked revision] | [unchecked revision] |
Line 111: | Line 111: | ||
The information dependence between the layers of the graph can be stated as follows. A one-dimensional FFT depends on the results of an earlier FFT in a perpendicular direction (which was performed at a previous layer) only if the one-dimensional sections processed by these FFTs intersect each other. Consequently, each <math>FFT_{i,y,k},~i=1,...,N</math>, with respect to the second coordinate depends on the results of each <math>FFT_{x,j,k},~j=1,...,N</math>, w.r.t. the first coordinate if and only if these transformations are executed in the same plane <math>k=K</math>. Accordingly, each <math>FFT_{i,j,z},~j=1,...,N</math>, w.r.t. the third coordinate depends on each <math>FFT_{i,y,k},~k=1,...,N</math>, w.r.t. the second coordinate if they belong to the same plane <math>i=I</math>. | The information dependence between the layers of the graph can be stated as follows. A one-dimensional FFT depends on the results of an earlier FFT in a perpendicular direction (which was performed at a previous layer) only if the one-dimensional sections processed by these FFTs intersect each other. Consequently, each <math>FFT_{i,y,k},~i=1,...,N</math>, with respect to the second coordinate depends on the results of each <math>FFT_{x,j,k},~j=1,...,N</math>, w.r.t. the first coordinate if and only if these transformations are executed in the same plane <math>k=K</math>. Accordingly, each <math>FFT_{i,j,z},~j=1,...,N</math>, w.r.t. the third coordinate depends on each <math>FFT_{i,y,k},~k=1,...,N</math>, w.r.t. the second coordinate if they belong to the same plane <math>i=I</math>. | ||
+ | |||
+ | |||
+ | === Parallelization resource of the algorithm === | ||
+ | |||
+ | One can distinguish at least two levels of parallelism in the above algorithm. In the first place, the execution of each one-dimensional FFT can be distributed between computational cores. | ||
+ | Second, at each stage of the algorithm (that is, at each layer of the graph), one-dimensional FFTs are independent of each other and can be performed in parallel (coordinate parallelism). In order to compare the parallel complexity (under coordinate parallelization) and the serial one, we take the one-dimensional FFT and the entrywise updating of the array (stage 4 of the algorithm) as the basic operations. Then the serial algorithm consists of <math>6N^2</math> one-dimensional FFTs, executed sequentially, and <math>N^3</math> operations over individual array entries. As for the parallel algorithm, it performs 6 steps of one-dimensional FFTs and one step of entrywise modification. By expressing the macro operations introduced above in terms of elementary arithmetic operations, we conclude that the complexity of the serial algorithm is <math>6N^3(\text{log}_2N)+N^3</math> operations, whereas the complexity of the parallel algorithm is <math>6N(\text{log}_2N)+1</math> operations. It follows that, for large <math>N</math>, the ratio of the serial to parallel complexity tends to <math>\propto N^2(6\text{log}_2N \text{ln}2+\text{ln}2+5)</math>. |
Revision as of 19:29, 4 February 2016
Primary authors of this description:V.M.Stepanenko, E.V.Mortikov, Vad.V.Voevodin (section 2.2)
Contents
- 1 Properties and structure of the algorithm
- 1.1 General description of the algorithm
- 1.2 Mathematical description of the algorithm
- 1.3 Computational kernel of the algorithm
- 1.4 Macro structure of the algorithm
- 1.5 Implementation scheme of the serial algorithm
- 1.6 Serial complexity of the algorithm
- 1.7 Information graph
- 1.8 Parallelization resource of the algorithm
1 Properties and structure of the algorithm
1.1 General description of the algorithm
The Poisson equation for the multidimensional space has the form [math] \sum_{i=1}^{N}\frac{\partial^2 \phi}{\partial x_i^2}=f,~\mathbf{x}\in D. [/math]
Here, [math]D \in \mathbb{R}^N[/math] is the domain in which the solution [math]\phi(\mathbf{x})[/math] is defined, and [math]\mathbf{x}=(x_1,...,x_N)^T[/math] is the vector of independent variables. The Poisson equation is supplemented by the boundary conditions [math] B(\phi)=F, \mathbf{x} \in \Gamma(D), [/math] where [math]\Gamma(D)[/math] is the boundary of [math]D[/math] and [math]B(\phi)[/math] is the operator defining the boundary conditions. The case [math]B(\phi)=\phi[/math] corresponds to the Dirichlet boundary condition, while [math]B(\phi)=\partial\phi/\partial n[/math], where [math]\mathbf{n}[/math] is the outer normal to the boundary [math]\Gamma(D)[/math], corresponds to the Neumann boundary condition. Sometimes mixed boundary conditions [math]B(\phi)=C\phi+\partial\phi/\partial n[/math], where [math]C[/math] is a constant, are also used. The so-called "periodic boundary conditions" may also occur. In this case, the problem is posed on an unbounded domain, but the solution is assumed to be periodic with respect to a subset of variables from [math]\mathbf{x}[/math].
The Poisson equation emerges in many problems of mathematical physics, for instance, in electrostatics (in this case, [math]\phi[/math] is the potential of the electric force) and hydrodynamics ([math]\phi[/math] is the pressure of a fluid or a gas). The parameter [math]N[/math] is 2 and 3 for the plane and three-dimensional problems, respectively.
The analytical form of the solution to the Poisson equation is not known in the case where the right-hand side is arbitrary and the boundary conditions are inhomogeneous. Consequently, in most applications, this equation is solved numerically. The most common discretization of the Poisson equation has the form
[math] \sum_{i=1}^{N}\frac{\phi_{k_1,...,k_i+1,...,k_N}-2\phi_{k_1,...,k_i,...,k_N}+\phi_{k_1,...,k_i-1,...,k_N}}{\Delta x_i^2}=f_{k_1,...,k_N},~(k_1,...,k_N) \in D_N. [/math]
Here, the second derivatives are replaced by second-order finite difference approximations (which creates the cross stencil for the plane problem), and the solution is sought on a discrete subset [math]D_N[/math] of the [math]N[/math]-dimensional space. The boundary conditions are also approximated by finite differences.
1.2 Mathematical description of the algorithm
Here, we examine a finite difference scheme for the most common problem related to the Poisson equation in the three-dimensional space:
[math] \frac{\phi_{i+1,j,k}-2\phi_{i,j,k}+\phi_{i-1,j,k}}{ \Delta x^2}\,+\, \frac{\phi_{i,j+1,k}-2\phi_{i,j,k}+\phi_{i,j-1,k}}{ \Delta y^2}\,+\, \frac{\phi_{i,j,k+1}-2\phi_{i,j,k}+\phi_{i,j,k-1}}{ \Delta z^2} = f_{i,j,k},~(i,j,k) \in D_h, [/math]
where [math]D_h=\{0:N_x-1\}\times\{0:N_y-1\}\times\{0:N_z-1\} [/math] is a parallelepiped in the grid domain. For simplicity, we impose the so-called 3-D periodic boundary conditions
[math] \begin{align} \phi_{0,j,k} &= \phi_{N_x,j,k},\\ \phi_{i,0,k} &= \phi_{i,N_y,k},\\ \phi_{i,j,0} &= \phi_{i,j,N_z}. \end{align} [/math]
The periodic boundary conditions are automatically satisfied if the solution is represented via the conventional discrete inverse Fourier transform:
[math] \phi_{i,j,k}=\frac{1}{N_x N_y N_z}\sum_{l=0}^{N_x-1}\sum_{m=0}^{N_y-1}\sum_{n=0}^{N_z-1}\Phi_{l,m,n}e^{2\pi \overline{i} \left(\frac{il}{N_x}+\frac{jm}{N_y}+\frac{kn}{N_z}\right)}. [/math]
Here, [math]\overline{i}=\sqrt{-1}[/math]. A similar representation is applied to the right-hand side [math]f_{i,j,k}[/math]. The convenience of using the Fourier transform for solving the discrete Poisson equation consists in the fact that the basis functions of the Fourier expansion are eigenfunctions of the discrete Laplace operator. Namely, by substituting the Fourier expansions of the unknown function [math]\phi_{i,j,k}[/math] and the right-hand side [math]f_{i,j,k}[/math] into the original equation, we obtain
[math] \Phi_{l,m,n}=-\frac{F_{l,m,n}}{4\left[\sin^2\left(\frac{\pi l}{N_x}\right) + \sin^2\left(\frac{\pi m}{N_y}\right) + \sin^2\left(\frac{\pi n}{N_z}\right) \right]}, [/math]
where [math]F_{l,m,n}[/math] is the Fourier transform of the right-hand side. This makes obvious the algorithm for solving the equation: first, the right-hand side is expanded into the Fourier series, then the above formula is used for calculating the Fourier coefficients of the solution; finally, the solution is reconstructed by applying the inverse Fourier transform.
1.3 Computational kernel of the algorithm
The one-dimensional Fourier transform is the computational kernel of this algorithm. Indeed, the discrete inverse Fourier transform can be written as
[math] \phi_{i,j,k}=\frac{1}{N_x} \sum_{l=0}^{N_x-1} \left[ e^{2\pi \overline{i}\left(\frac{il}{N_x}\right)} \frac{1}{N_y} \sum_{m=0}^{N_y-1} \left[ e^{2\pi \overline{i}\left(\frac{jm}{N_y}\right)} \frac{1}{N_z} \sum_{n=0}^{N_z-1} \Phi_{l,m,n}e^{2\pi \overline{i} \left(\frac{kn}{N_z}\right)}\right]\right]. [/math]
One can see that the three-dimensional Fourier transform reduces to the sequence of three one-dimensional transforms. A widely used tool for calculating the one-dimensional transform is an efficient algorithm called the fast Fourier transform (FFT) [1].
1.4 Macro structure of the algorithm
From the above discussion, it is clear that the fast Fourier transform is the basic macro operation in the algorithm for solving the Poisson equation. In what follows, we denote this operation by [math]\text{FFT}_i,~i=x,y,z[/math] in accordance with its direction, while the inverse Fourier transforms are denoted by [math]\text{IFFT}_i,~i=x,y,z[/math].
1.5 Implementation scheme of the serial algorithm
For brevity, we use the following notation for the grid functions: [math]\phi_h=\{\phi_{i,j,k},~(i,j,k) \in D_h\},~F_h=\{F_{l,m,n},~l=0,...,N_x-1,~m=0,...,N_y-1,~n=0,...,N_z-1\}[/math]. A similar notation is used for [math]f_h[/math] and [math]\Phi_h[/math]. Now, the algorithm can be written as follows:
- Calculate [math]\text{FFT}_x(f_h)[/math]
- Calculate [math]\text{FFT}_y(\text{FFT}_x(f_h))[/math]
- Calculate [math]\text{FFT}_z(\text{FFT}_y(\text{FFT}_x(f_h)))=F_h[/math]
- Find [math]\Phi_h[/math] from [math]F_h[/math]
- Calculate [math]\text{IFFT}_x(\Phi_h)[/math]
- Calculate [math]\text{IFFT}_y(\text{IFFT}_x(\Phi_h))[/math]
- Calculate [math]\text{IFFT}_z(\text{IFFT}_y(\text{IFFT}_x(\Phi_h)))=\phi_h[/math]
It is remarkable that the entire algorithm can be implemented with the use of a single three-dimensional array of size [math]N_x \times N_y \times N_z[/math]. Indeed, the results of one-dimensional Fourier transforms in one direction can be written to the input array, and the resulting array can be used as an input for transforms in the next direction. Operation (4) is an entrywise array modification.
1.6 Serial complexity of the algorithm
If the domain [math]D_h[/math] is a cube, then [math]N_x=N_y=N_z=N=2^k[/math]. Then the complexity of a one-dimensional FFT in any direction is [math]N(\text{log}_2 N)[/math] operations (in order). At each stage, excepting the fourth, one performs [math]N^2[/math] one-dimensional FFTs, while the number of operations at stage 4 is [math]N^3[/math] (again in order). Thus, the overall number of arithmetic operations is [math]N^3(6\text{log}_2 N+1)[/math].
1.7 Information graph
We present the information graph only for stages 1-4 because stages 5-7 are implemented analogously to stages 1-3.
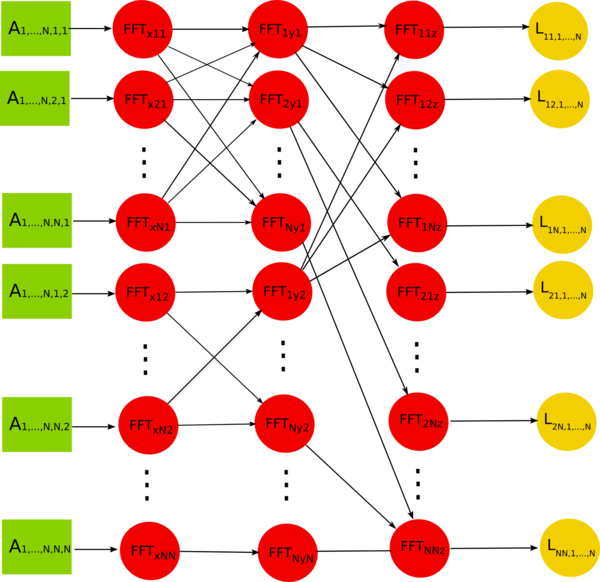
The information dependence between the layers of the graph can be stated as follows. A one-dimensional FFT depends on the results of an earlier FFT in a perpendicular direction (which was performed at a previous layer) only if the one-dimensional sections processed by these FFTs intersect each other. Consequently, each [math]FFT_{i,y,k},~i=1,...,N[/math], with respect to the second coordinate depends on the results of each [math]FFT_{x,j,k},~j=1,...,N[/math], w.r.t. the first coordinate if and only if these transformations are executed in the same plane [math]k=K[/math]. Accordingly, each [math]FFT_{i,j,z},~j=1,...,N[/math], w.r.t. the third coordinate depends on each [math]FFT_{i,y,k},~k=1,...,N[/math], w.r.t. the second coordinate if they belong to the same plane [math]i=I[/math].
1.8 Parallelization resource of the algorithm
One can distinguish at least two levels of parallelism in the above algorithm. In the first place, the execution of each one-dimensional FFT can be distributed between computational cores.
Second, at each stage of the algorithm (that is, at each layer of the graph), one-dimensional FFTs are independent of each other and can be performed in parallel (coordinate parallelism). In order to compare the parallel complexity (under coordinate parallelization) and the serial one, we take the one-dimensional FFT and the entrywise updating of the array (stage 4 of the algorithm) as the basic operations. Then the serial algorithm consists of [math]6N^2[/math] one-dimensional FFTs, executed sequentially, and [math]N^3[/math] operations over individual array entries. As for the parallel algorithm, it performs 6 steps of one-dimensional FFTs and one step of entrywise modification. By expressing the macro operations introduced above in terms of elementary arithmetic operations, we conclude that the complexity of the serial algorithm is [math]6N^3(\text{log}_2N)+N^3[/math] operations, whereas the complexity of the parallel algorithm is [math]6N(\text{log}_2N)+1[/math] operations. It follows that, for large [math]N[/math], the ratio of the serial to parallel complexity tends to [math]\propto N^2(6\text{log}_2N \text{ln}2+\text{ln}2+5)[/math].
- ↑ Марчук Г.И. Методы вычислительной математики, М., 1977, 456с.